
A new device could help us understand how AI makes decisions.
Scientists from Penn State, US have developed what's essentially an electronic tongue that can distinguish tastes better than humans.
They hope it could 'revolutionise' the way we detect chemical and environmental changes with potential applications in medical diagnostics and even spotting spoiled food.
Advert
According to the paper published in the journal Nature, the graphene-based device's sensor can effectively detect and classify different substances, while also evaluating their 'quality, authenticity and freshness.'
The team hopes this kind of information can help address the current black box problem in AI.
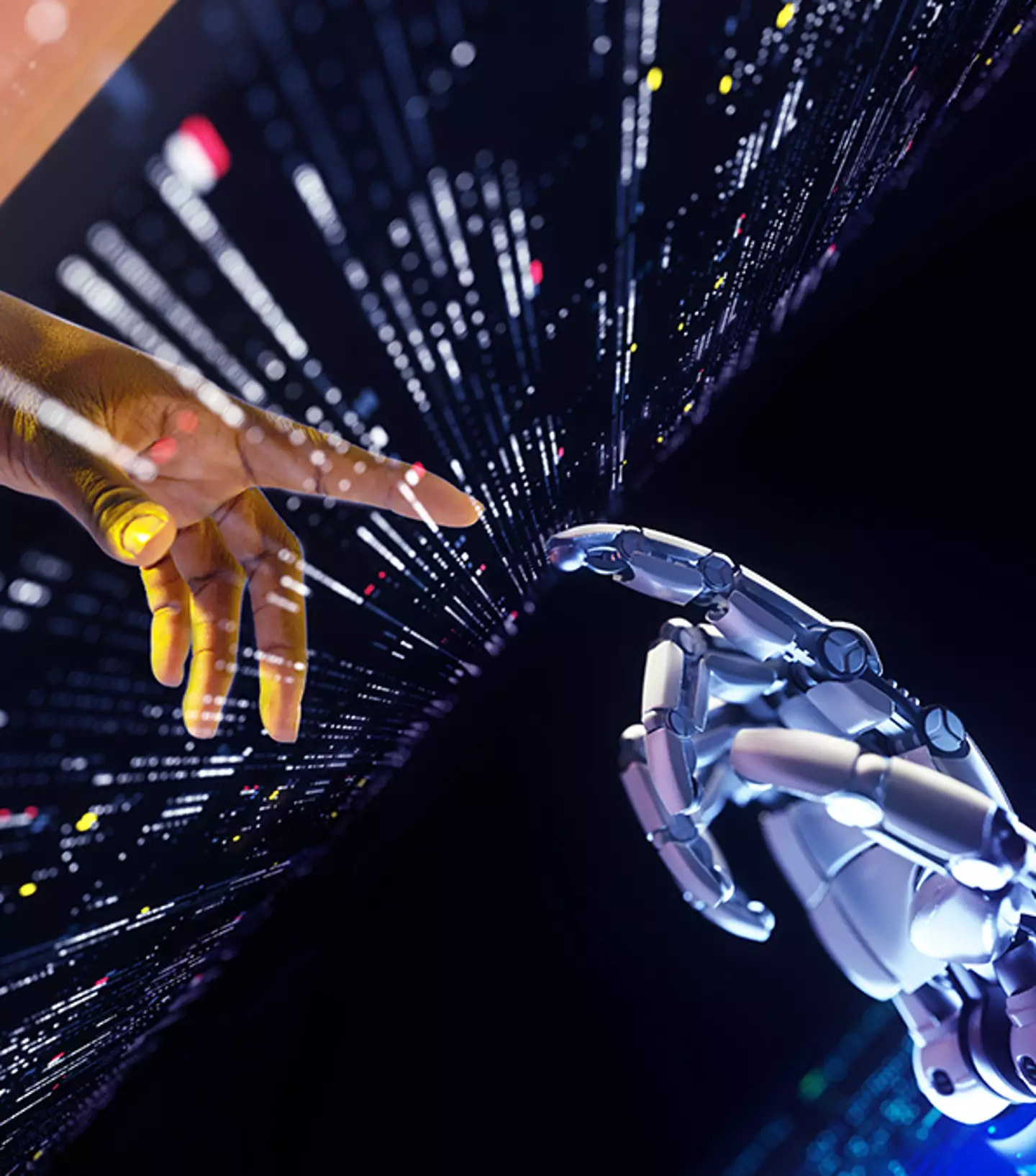
If you aren't familiar with it, the black box problem is our lack of understanding of how AI systems arrive at their decisions and allow certain outcomes.
Advert
But with this kind of technology, we can start to uncover the 'inner thoughts' of AI.
The team achieved this by reverse engineering how the neural network determined differences between different beverages like milk, coffee and fizzy drinks.
“We’re trying to make an artificial tongue, but the process of how we experience different foods involves more than just the tongue,” explained Saptarshi Das, a professor of engineering science and mechanics at Penn State.
With this data, the researchers discovered a 'glimpse into the neural network’s decision-making process' - which they claimed could lead to improvements in AI safety and development.
Advert
“We have the tongue itself, consisting of taste receptors that interact with food species and send their information to the gustatory cortex — a biological neural network.”
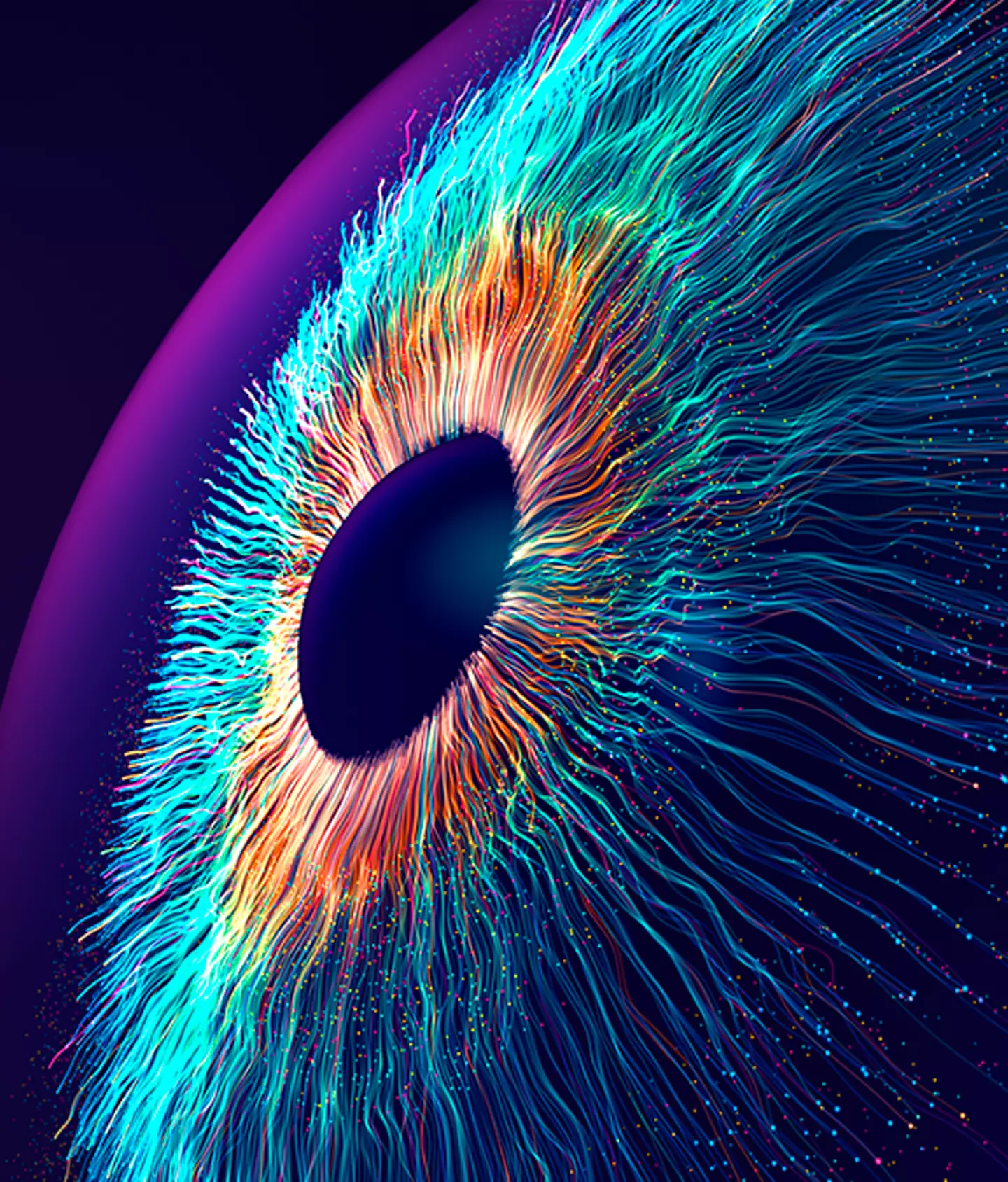
This part of the brain helps us to perceive and interpret various tastes and primarily has five broad categories sweet, sour, bitter, salty and savoury.
The team found that the electronic tongue achieved more than 95% accuracy in tasting when tested against human-selected parameters.
Advert
Interestingly, the findings were a result of something called Shapley additive explanations.
This basically means that the neural network focused on the data it deemed most important for identifying different tastes rather than the parameters set by humans.
“We found that the network looked at more subtle characteristics in the data – things we, as humans, struggle to define properly,” Professor Das added.
“And because the neural network considers the sensor characteristics holistically, it mitigates variations that might occur day-to-day. In terms of the milk, the neural network can determine the varying water content of the milk and, in that context, determine if any indicators of degradation are meaningful enough to be considered a food safety issue.”